Note
Go to the end to download the full example code.
Discrepencies with StandardScaler¶
A StandardScaler does
a very basic scaling. The conversion in ONNX assumes that
(x / y)
is equivalent to x * ( 1 / y)
but that’s not
true with float or double (see
Will the compiler optimize division into multiplication).
Even if the difference is small,
it may introduce discrepencies if the next step is
a decision tree. One small difference and the decision
follows another path in the tree. Let’s see how to solve
that issue.
An example with fails¶
This is not a typical example, it is build to make it fails
based on the assumption (x / y)
is usually different from
x * ( 1 / y)
on a computer.
import onnxruntime
import onnx
import os
import math
import numpy as np
import matplotlib.pyplot as plt
from onnx.tools.net_drawer import GetPydotGraph, GetOpNodeProducer
from onnxruntime import InferenceSession
from sklearn.datasets import make_regression
from sklearn.model_selection import train_test_split
from sklearn.pipeline import Pipeline
from sklearn.preprocessing import StandardScaler
from sklearn.tree import DecisionTreeRegressor
from skl2onnx.sklapi import CastTransformer
from skl2onnx import to_onnx
The weird data.
X, y = make_regression(10000, 10, random_state=3)
X_train, X_test, y_train, _ = train_test_split(X, y, random_state=3)
Xi_train, yi_train = X_train.copy(), y_train.copy()
Xi_test = X_test.copy()
for i in range(X.shape[1]):
Xi_train[:, i] = (Xi_train[:, i] * math.pi * 2**i).astype(np.int64)
Xi_test[:, i] = (Xi_test[:, i] * math.pi * 2**i).astype(np.int64)
max_depth = 10
Xi_test = Xi_test.astype(np.float32)
A simple model.
Conversion into ONNX.
onx1 = to_onnx(model1, X_train[:1].astype(np.float32), target_opset=15)
sess1 = InferenceSession(onx1.SerializeToString(), providers=["CPUExecutionProvider"])
And the maximum difference.
322.39065126389346
The graph.
pydot_graph = GetPydotGraph(
onx1.graph,
name=onx1.graph.name,
rankdir="TB",
node_producer=GetOpNodeProducer(
"docstring", color="yellow", fillcolor="yellow", style="filled"
),
)
pydot_graph.write_dot("cast1.dot")
os.system("dot -O -Gdpi=300 -Tpng cast1.dot")
image = plt.imread("cast1.dot.png")
fig, ax = plt.subplots(figsize=(40, 20))
ax.imshow(image)
ax.axis("off")
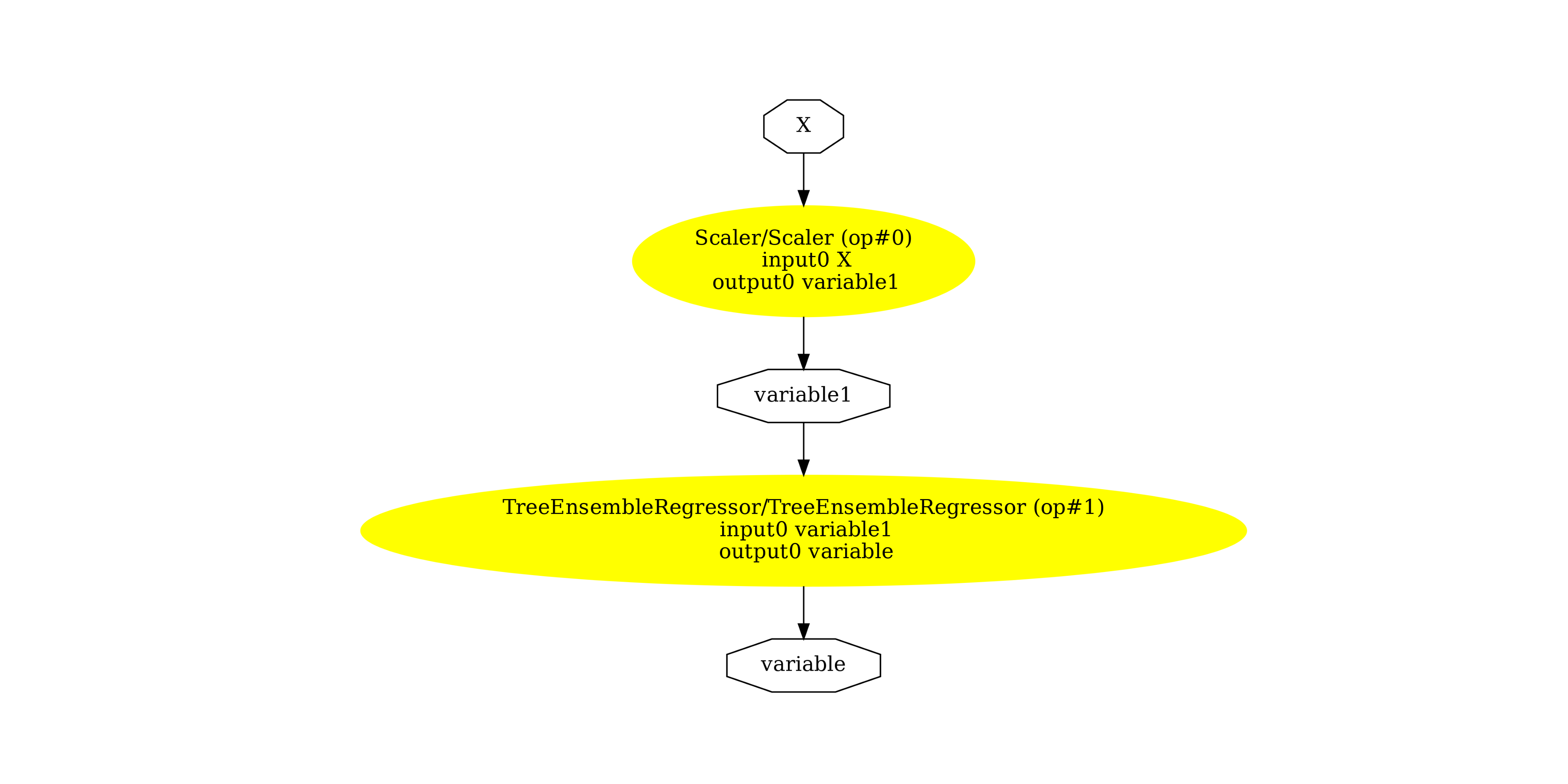
(-0.5, 2536.5, 1707.5, -0.5)
New pipeline¶
Fixing the conversion requires to replace (x * (1 / y)
by (x / y)
and this division must happen in double.
By default, the sklearn-onnx assumes every
computer should happen in float. ONNX 1.7 specifications
does not support double scaling (input and output does,
but not the parameters). The solution needs to
change the conversion (remove node Scaler by using option
‘div’) and to use double by inserting an explicit
Cast.
model2 = Pipeline(
[
("cast64", CastTransformer(dtype=np.float64)),
("scaler", StandardScaler()),
("cast", CastTransformer()),
("dt", DecisionTreeRegressor(max_depth=max_depth)),
]
)
model2.fit(Xi_train, yi_train)
exp2 = model2.predict(Xi_test)
onx2 = to_onnx(
model2,
X_train[:1].astype(np.float32),
options={StandardScaler: {"div": "div_cast"}},
target_opset=15,
)
sess2 = InferenceSession(onx2.SerializeToString(), providers=["CPUExecutionProvider"])
got2 = sess2.run(None, {"X": Xi_test})[0]
md2 = maxdiff(exp2, got2)
print(md2)
2.9884569016758178e-05
The graph.
pydot_graph = GetPydotGraph(
onx2.graph,
name=onx2.graph.name,
rankdir="TB",
node_producer=GetOpNodeProducer(
"docstring", color="yellow", fillcolor="yellow", style="filled"
),
)
pydot_graph.write_dot("cast2.dot")
os.system("dot -O -Gdpi=300 -Tpng cast2.dot")
image = plt.imread("cast2.dot.png")
fig, ax = plt.subplots(figsize=(40, 20))
ax.imshow(image)
ax.axis("off")
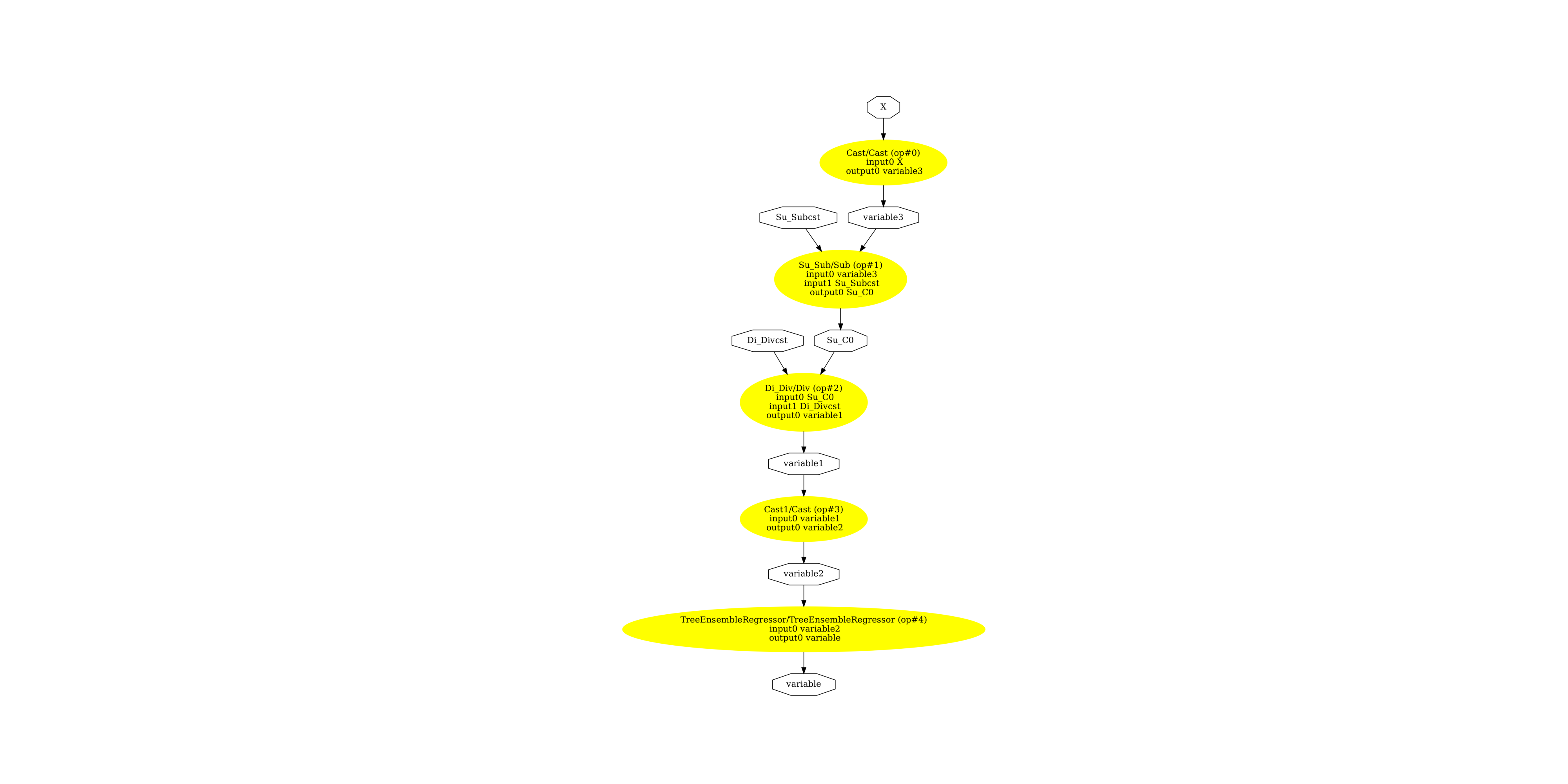
(-0.5, 2536.5, 4171.5, -0.5)
Versions used for this example
import sklearn # noqa
print("numpy:", np.__version__)
print("scikit-learn:", sklearn.__version__)
import skl2onnx # noqa
print("onnx: ", onnx.__version__)
print("onnxruntime: ", onnxruntime.__version__)
print("skl2onnx: ", skl2onnx.__version__)
numpy: 1.26.4
scikit-learn: 1.6.dev0
onnx: 1.17.0
onnxruntime: 1.18.0+cu118
skl2onnx: 1.17.0
Total running time of the script: (0 minutes 4.086 seconds)